
AI is an incredibly powerful tool, but with great power comes great responsibility. I’ve seen far too many researchers stumble—sometimes even ruin their careers—by making avoidable mistakes when using AI. I don’t want you, our beloved reader, to fall into the same trap. That’s why I’ve put together this list of the top pitfalls to watch out for when incorporating AI into your work as a researcher.
1. Copyright Complications
The first—and probably most important—risk is copyright. In the Western world, particularly in the U.S., courts have ruled that AI-generated content cannot be copyrighted by the prompter. Simply put, if you write a prompt in an AI tool like ChatGPT, you don’t own the output.
This means anything AI generates—text, music, images—is in the public domain. While you can use it, so can everyone else. It’s a free for all! This has significant implications for researchers, especially when it comes to writing. Copyright doesn’t protect ideas, but it does protect the words you use to express them. So, be cautious when using AI to help draft papers or write books, as you won’t technically have ownership over the generated content.
To complicate things further, different countries are taking different approaches. For example, China is now granting copyright to the prompter in most cases, meaning the rules around AI ownership can vary significantly depending on where you are.
Who doesn’t love a legal spaghetti bowl?
2. Privacy and Confidentiality Risks
A lesser-known pitfall is that most AI tools—by default—allow themselves to use whatever you input for training purposes. This means if you upload a draft of your super-duper novel paper, that content can be swallowed and embedded into the AI’s monstrous “brain” and could resurface elsewhere without your knowledge or consent. Someone across the world could ask a related question, and suddenly parts of your unpublished work are showing up in their results.
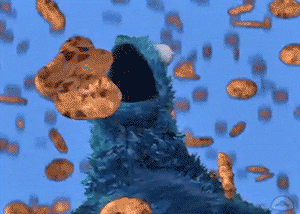
The lesson here? Don’t put anything into AI that’s copyrighted, confidential, or hasn’t been published yet. And on that note, never use AI for peer review. Running someone’s unpublished research through an AI is a double no-no—you don’t own the copyright, and the work is still confidential.
This kind of breach has already caused a scandal. In Australia, reviewers of major research grants used ChatGPT to help write their reviews, which became national news, and people got into serious trouble. Keep those peer reviews private, and don’t let AI anywhere near them.
3. AI Isn’t a Truth Machine
A major pitfall is trusting AI as a truth machine. These tools are just sophisticated statistical models predicting the next likely word—they sound intelligent, but they’re not. And they get things wrong. Sometimes, comically wrong.
For example, a Washington Post fact-check study found that ChatGPT is wrong about 10% of the time. And while AI is improving, it’s still far from perfect. Don’t rely on it for facts and references, because it’s not built to give accurate information—it’s built to generate plausible-sounding text.
One particularly hilarious example came from Google’s AI-generated search summaries. People typed in “smoking while pregnant,” and the AI confidently recommended two to three cigarettes per day. It also advised someone to use non-toxic glue for pizza toppings that wouldn’t stick and suggested geologists recommend eating one small rock per day. These absurd mistakes happened because AI can’t always distinguish between legitimate sources and, say, an April Fool’s joke.
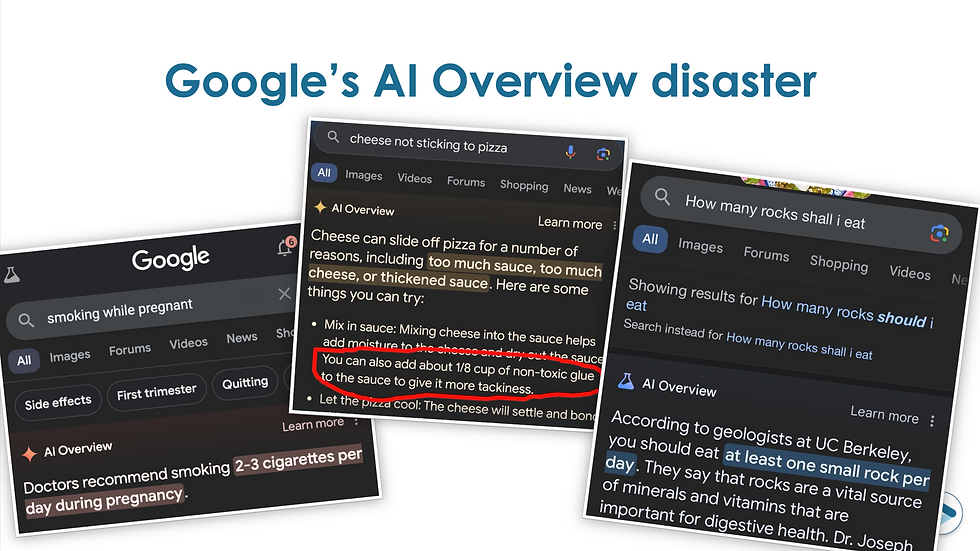
In my last blog post, I explored this issue in relation to AI-generated images, which suffer from even more serious inaccuracy issues.
4. Bias in AI Outputs
Bias is another issue. For instance, I asked an AI to create an image of researchers, and everyone in the picture was white. Is AI racist? No, AI doesn’t have ethics or preferences. The problem lies in the training data. If the AI was trained on images dominated by white researchers, it will continue to produce that bias.
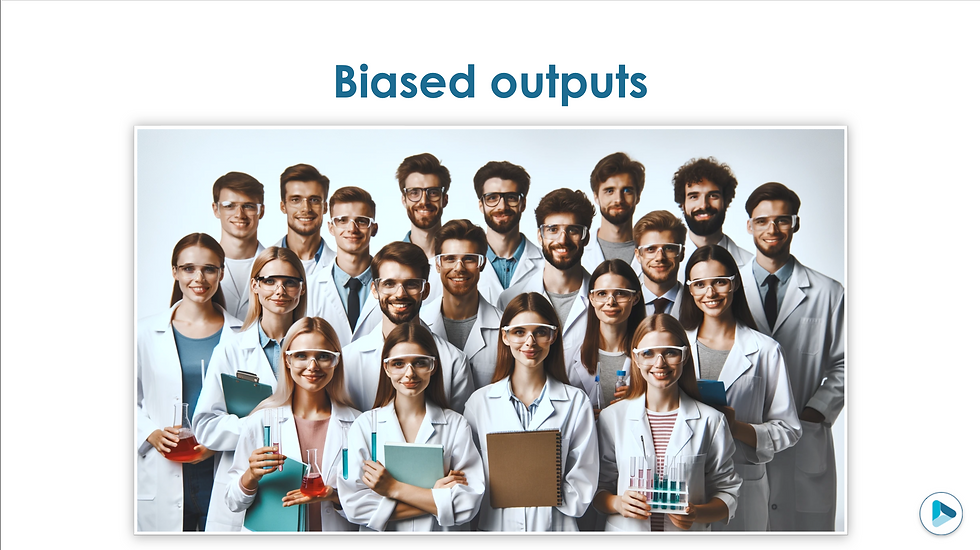
This bias can manifest in other ways too. In an infamous incident, Google tried to counteract the tendency of its AI to generate images dominated by white people. However, the adjustment went too far. As a result, when users asked for historically accurate figures, such as German soldiers from World War II, the AI generated Black and Asian women—clearly inaccurate. It even produced images like a Black Hillary Clinton, a Black Elon Musk, and an all-Black team of the founding fathers of the United States.

These biases are baked into the system based on the data used to train the AI, and you can inadvertently perpetuate these biases if you’re not careful.
Conclusion
AI is a powerful tool, but it’s important to tread carefully. Don’t fall into the pitfalls of copyright misuse, exposing confidential material, relying on it for factual accuracy, or reinforcing biases. AI can be a powerful sidekick, but it needs your guidance to avoid these traps.
Thank you for reading, and stay tuned for more on this topic. This is just the second in a series of articles I’m planning to write about AI and science communication. If you’re interested in learning more, I also run workshops on AI for science communication. Whether in person or online, I’d love to bring this workshop to your institution. Just follow this link to get in touch.
Stay curious, and keep creating!
コメント